Talents are everywhere, but opportunities are not.
Nearly 220 million individuals, with a median age of 17, live in Nigeria, making it the most populous country in Africa. Some of the most critical problems facing the country include the high rate of unemployment, the high rate of poverty, little or no access to electricity, and costly access to the internet. While these challenges make it more difficult to access the world through digital devices, especially for learning critical digital skills, the youth of Nigeria have largely embraced technology, creating a massive talent opportunity just waiting to be unlocked. Data Scientists Network (DSN), formerly known as Data Science Nigeria, is an organization focused on doing just that and is working to support the development of one million Artificial Intelligence (AI) talents who can catalyze solution-oriented innovations for emerging market.
For the last five years, DSN has been working hard and intentionally to expand access to AI education and expertise within the field, regardless of socio-economic status and resource challenges of the continent. For instance, DSN has continued to explore context-aware and homegrown approaches to overcome lack of internet, power, digital devices, and AI expertise in Africa. DSN’s model is an inclusive one with large-scale AI knowledge transfer through community learning groups, offline learning contents, a global network of mentors from the first world, hands-on project-based learning, and direct deployment through project participation and job support.
DSN creates communities of AI learners all around Nigeria, providing grants for internet access, devices, and electricity. These communities meet weekly, where they can solve problems and learn from one other. Through this process, DSN has created about 100 vibrant learning communities across Nigeria. These communities are trained by our global network of mentors, which includes top AI professionals, AI startup founders, and some DSN Alumni, who are based in the US, UK, Canada and Europe. Many of these interactions are recorded and made available online and offline. For offline use, these videos are aggregated and stored in a physical storage device called the AI Knowledge Box. This resource is duplicated, and a copy is shared with each community to ensure they have access to world-class AI knowledge offline—anywhere and anytime they want. DSN also supports world-class events where participants are engaged through hands-on, project-based activities and gain real-world problem-solving skills in the process. Additionally, DSN absorbs top talents into its talent pool, supporting and mentoring the development of job-ready candidates in the process.
DSN’s unique approach has secured global recognitions. The World Economic Forum has recognized DSN for its dynamic approach to education in Africa. UNESCO Science Report has also recognized DSN’s model as one of the best ways of learning outside the four walls of a university. Thus far, DSN has trained over 500,000 individuals, created about 100 local communities, and extended AI introductory knowledge to over 3 million people through its free learning contents. DSN has quickly grown into the number one AI learning community and solution delivery network in Africa—as well as one of the world’s largest communities of digitally savvy people. DSN was recognized for this outstanding community-building achievement when it received the Matthai Impact Award at Deep Indaba. DSN has received several other accolades and recognitions by several governments and organizations. Collectively, these serve as a testament to the effectiveness of DSN’s model. Some of the awards are listed below.
- IRCAI UNESCO – Listed in the IRCAI/UNESCO Top 100 AI products in 2021 – with two products listed for solving sustainable development goals – https://bit.ly/IRCAI_2021-Report
- Economic and Computation Conference 2021 award – The best academic poster at ACM Conference on Economics and Computation (EC’ 20) – https://bit.ly/DSN-Best-Postal-Award_GCEC2020
- XPrize Award 2021 – The only African finalist in the competition on building advanced AI algorithms to address COVID19 – https://bit.ly/XPRIZEPress
There is a well-known African proverb that goes—”A tree doesn’t make a forest.” Likewise, the successes cultivated throughout DSN would simply not have been possible on its own. These have been achieved because of many strong partnerships and collaborations. Of note is DSN’s partnership with NVIDIA to enhance the application of knowledge and use cases, deliver additional learning content, and provide further international exposure and hands-on participation in project-based training. One such project was the Hands-on Project-based Data Visualization Masterclass organized by DSN in partnership with NVIDIA, which brought together more than 850 people online and about 100 people in person. Another such partnership was the Free AI Classes in Every City, which was held in over 120 locations in Nigeria and multiple locations in Kenya and Uganda. It is an educationally rich, five-day program that introduces participants into the world of AI. Over 7,500 people participated in the learning and then a hackathon was organized that tested the participants’ capacity. The top 400 participants were selected to receive additional learning materials provided by NVIDIA.
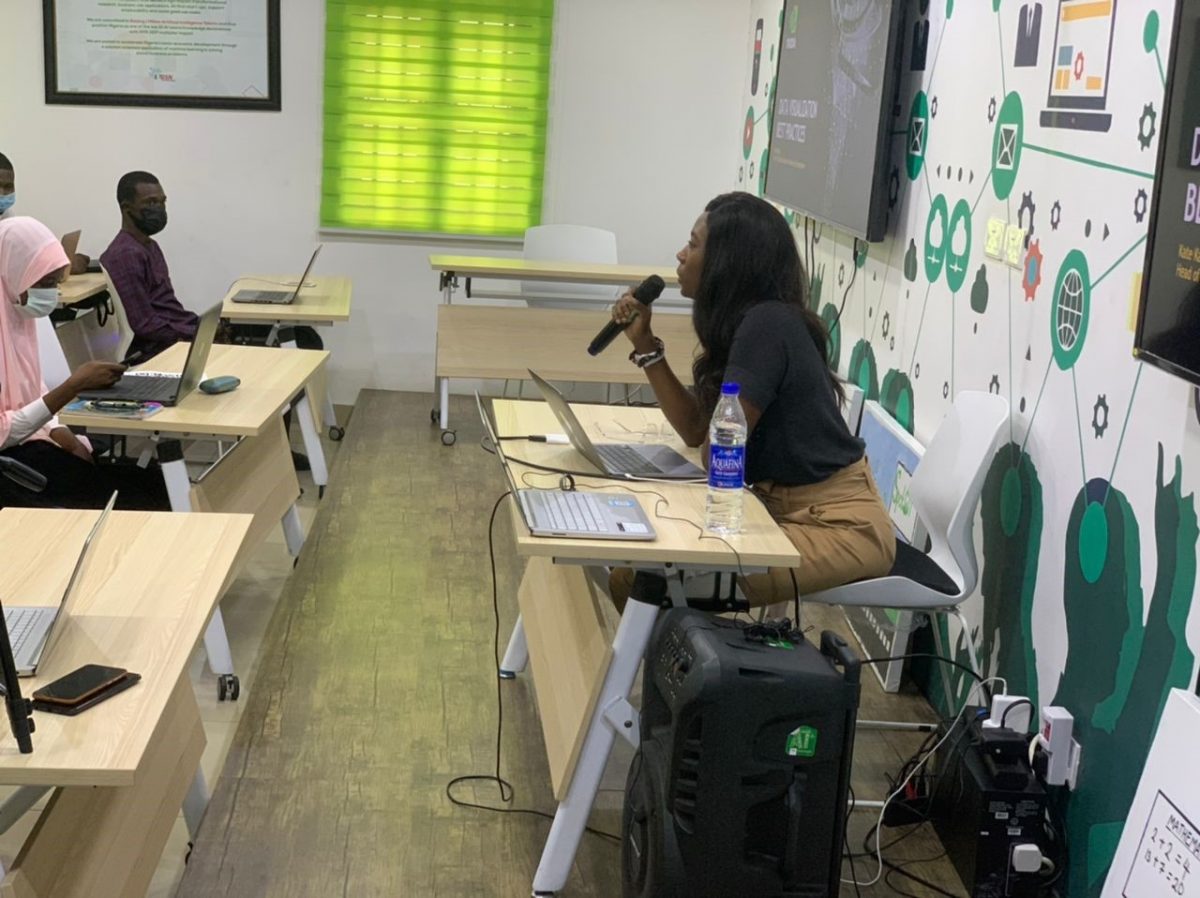
NVIDIA has been supporting DSN’s AI democratization agenda by donating workstations and other hardware like the Jetson Nano. Participation in knowledge sessions has also been supported through this partnership. For instance, in one such session, the DSN community was introduced to NVIDIA’s Omniverse. The Omniverse, much like the metaverse, is a real-time graphics collaboration platform created by NVIDIA. It has been used for applications in the visual effects and digital twin industrial simulation space. Apart from the Omniverse, NVIDIA products like CUDA were also introduced to the DSN community. NVIDIA has also been hands-on about project participation. In partnership with DSN and EFINA on a session focused on a Financial Inclusion Dashboard, participants learned how to build interactive dashboards using Power BI. This activity helped participants with real-life case studies and made them more job ready as well. Through special tickets to NVIDIA premium classes, DSN community members were placed at the forefront of cutting-edge technology and direct access to world-renowned AI professionals. NVIDIA was also involved in mentoring a DSN incubation programs for young local AI innovators and startups, providing free GCP credit units for the selected “techpreneurs”. These efforts, among many others, have significantly enriched the educational impact for so many students, demonstrating that great collaborations can move mountains.
With NVIDIA’s strategic partnership, DSN has been able to scale its outreach and widen its capacity-building portfolio. 400 DSN community members are part of the NVIDIA DLI program, while 200 will be DLI ambassadors. Also, during the partnership, DSN has opened ten new communities, increasing the total number of DSN Communities to nearly 100. DSN has also extended beyond the shores of Nigeria, building communities in Kenya and Uganda, with Ghana, Tunisia, and South Africa in the pipeline. This partnership has greatly expanded DSN’s ability to deliver learning to many young Africans, irrespective of their constrained background or lack of access to learning infrastructure. With this collaboration, DSN is able to leave a lasting and meaningful impact on many, many lives.
With these successes, DSN and NVIDIA are well positioned to explore more opportunities, such as embedding DLI classes into the university education in Nigeria, standardizing Nigeria’s learning curriculum, and using NVIDIA’s platform to accelerate research for the developer community, student ecosystem, startups, and universities. To further scale up, DSN is also exploring the possibilities of having NVIDIA’s workstations across centres of excellence throughout Nigeria’s universities. DSN and NVIDIA are looking to continue giving DLI materials to DSN’s outstanding community members, providing access to the cutting edge of learning. With this, younger, disadvantaged Africans can pick the right digital skill and translate the knowledge to the next set of people through mentorship. Therefore, through this continued cycle, DSN and NVIDIA are creating great momentum in advancing the next generation of AI and data science talent to lead the fourth industrial revolution.
